In recent years generative models based on generative adversarial networks GANs 11 and variational autoencoders VAEs 17 have been widely. Generative Models for Automatic Chemical Design.
Pdf Generative Models For Automatic Chemical Design Semantic Scholar
We begin by revisiting early inverse design algorithms.
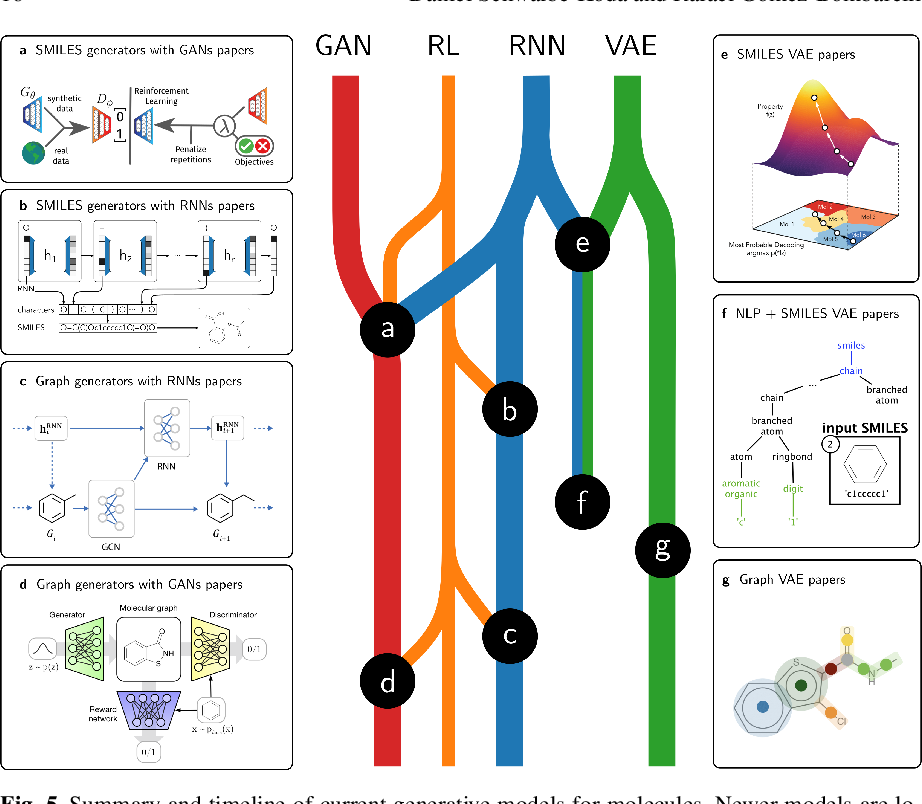
. The de novo design of molecular structures using deep learning generative models introduces an encouraging solution to drug discovery in the face of the continuously increased cost of new drug development. AU - Adriaenssens Sigrid. Materials discovery is decisive for tackling urgent challenges related to energy the environment health care and many others.
Continuous representations of molecules allow us to automatically generate novel chemical structures by performing simple operations in the latent space such as decoding random vectors perturbing known chemical structures or interpolating between molecules. From the generation of original texts images and videos to the scratching of novel molecular structures the incredible creativity of deep. T1 - Machine learning generative models for automatic design of multi-material 3D printed composite solids.
Generative models produce large numbers of candidate molecules and the physical realizations of these candidates will. Recently deep generative neural networks have become a very active research frontier in de novo drug discovery both in theoretical and in experimental evidence shedding light on a promising new. In chemistry conventional methodologies for innovation usually rely on expensive and incremental strategies to optimize properties from.
This work is partially supported by the Princeton Catalysis Initiative at Princeton University. Then we introduce generative models for molecular systems and categorize them according to their architecture and molecular representation. And Müller K.
We begin by revisiting early inverse design algorithms. The original scheme featuring Bayesian optimization over the latent space of a variational autoencoder suffers from the pathology. Generative Models for Automatic Chemical Design.
Current generative models have been successful in learning and generating novel data from different types of real. One such neural network model JT-VAE the Junction Tree Variational Auto-Encoder excels at. In this chapter we examine the way in which current deep generative models are addressing the inverse chemical discovery paradigm.
We begin by revisiting early inverse design algorithms. Daniel Schwalbe-Koda Rafael Gómez-Bombarelli. Generative Models for Automatic Chemical Design Submitted by dskoda on Sat 2020-06-06 1712 D.
In principle this method of converting from a molecular representation to a. The model described in the paper Automatic. Overwhelming evidence has been accumulating that materials informatics can provide a novel solution for materials discovery.
Generative Models for Automatic Chemical Design. AU - Menguc Yigit. A variety of so-called generative deep learning models have.
On the other hand inverse approaches map properties to structures thus expediting the design of novel useful compounds. AU - Wallin Thomas J. Using this classification we review the evolution and performance of important molecular generation schemes reported in the literature.
AU - Chiaramonte Maurizio. Automated molecular design methods support medicinal chemistry by efficient sampling of untapped drug-like chemical space 1 2 3. Generative Models for Automatic Chemical Design in Machine Learning Meets Quantum Physics K.
Gómez-Bombarelli Published 2 July 2019 Computer Science ArXiv Materials discovery is decisive for tackling urgent challenges related to energy the environment health care and many others. Then we introduce generative models for molecular systems and categorize them according to their architecture and molecular representation. In the context of drug discovery graph convolutions have been applied to molecular property prediction 9495 and in generative models for de novo drug design 96.
Materials discovery is decisive for tackling urgent challenges related to energy the environment health care and many others. While the conventional approach to innovation relies mainly on experimentation the generative models stemming from the field of machine learning can realize the long-held dream of inverse design where properties are mapped to the. TitleGenerative Models for Automatic Chemical Design.
We use the research papers Automatic chemical design using a data-driven continuous representation of molecules and MolGAN. The tools for inverse design especially those stemming from the field of machine learning have shown rapid progress in the last several years and have allowed chemical space to be framed into probabilistic data-driven models. Schütt Chmiela S.
Schwalbe-Koda and Gómez-Bombarelli R. We have proposed a Transformer-encoder-based generative model for a de novo drug design and sample evaluation of the original pretrained model and the RL-optimized generative model shows that our method is superior to the existing generative models in terms of the percentage of valid compounds generated and the propertyactivity distribution of. Generative Models for Automatic Chemical Design D.
The original scheme featuring Bayesian optimization over the latent space of a variational autoencoder suffers from the pathology that it. Springer International Publishing 2020 pp. Recent advances in generative modelling allow designing novel compounds through deep neural networks.
N1 - Funding Information. 2122 We apply such generative models to chemical design using a pair of deep networks trained as an autoencoder to convert molecules. Generative Models for Automatic Chemical Design - CORE Reader.
Text20 speech and music2122 We apply such generative models to chemical design using a pair of deep networks trained as an autoencoder to convert molecules represented as SMILES strings into a continuous vector representation. Automatic Chemical Design is a framework for generating novel molecules with optimized properties. Automatic chemical design using generative models Gradient-based hyperparameter tuning Structured latent-variable models for modeling video and Convolutional networks on graphs.
AU - Xue Tianju.
Generative Models For Automatic Chemical Design Arxiv Vanity
Github Chennnnnyize Generative Molecules
Pdf Generative Models For Automatic Chemical Design Semantic Scholar
Generative Models For Molecular Discovery Recent Advances And Challenges Bilodeau Wires Computational Molecular Science Wiley Online Library
Pdf Generative Models For Automatic Chemical Design Semantic Scholar
Pdf Generative Models For Automatic Chemical Design Semantic Scholar
Generative Models For Automatic Chemical Design Arxiv Vanity
Pdf Generative Models For Automatic Chemical Design Semantic Scholar
0 comments
Post a Comment